How E-commerce Sites Predict Your Next Purchase
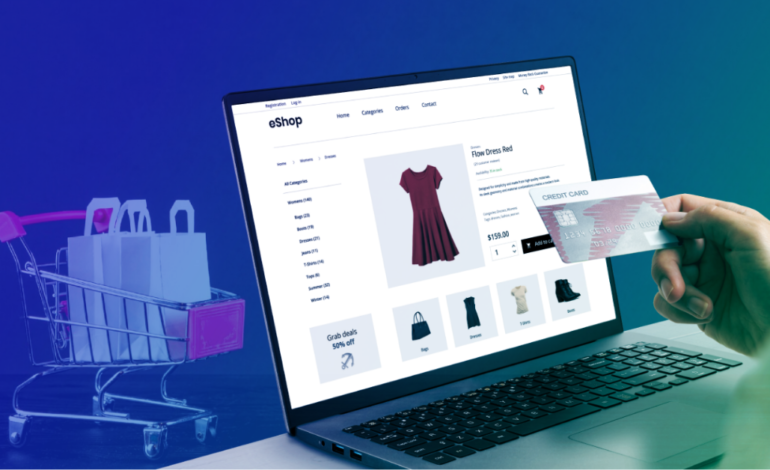
In the latest virtual age, e-commerce websites have become synonymous with convenience, supplying a large array of products at the click of a button. However, what sets aside the great e-trade structures from the rest is their capacity to provide customized hints tailor-made to every consumer’s preferences and surfing history.
Behind these seemingly intuitive hints lies a complicated web of algorithms designed to research statistics and expect consumer conduct. In this article, we delve into the technological know-how at the back of recommendation algorithms, exploring how e-commerce websites leverage statistics to count on your next buy.
Understanding Recommendation Algorithms
At the coronary heart of e-commerce advice structures are state-of-the-art algorithms that sift through massive quantities of statistics to identify styles and correlations. These algorithms employ various techniques, including collaborative filtering, content-based filtering, and hybrid methods, to generate personalized tips for users.
- Collaborative Filtering: This technique analyzes user behavior and options to discover similarities and styles amongst customers. By leveraging the information of the crowd, collaborative filtering algorithms recommend products that customers with similar tastes have favored or bought.
- Content-Based Filtering: Content-based filtering algorithms are conscious of the attributes of products and customers’ past interactions with similar objects. By studying product descriptions, consumer opinions, and different metadata, those algorithms advise gadgets that fit customers’ preferences and interests.
- Hybrid Approaches: Many e-trade structures integrate collaborative filtering and content material-based filtering techniques to enhance recommendation accuracy. By leveraging the strengths of both processes, hybrid advice structures can provide greater nuanced and correct recommendations.
The Data Behind Personalization
To energy advice algorithms, e-trade websites rely upon a wealth of statistics accrued from customers’ interactions with the platform. This information includes:
- Purchase History: Analyzing customers’ beyond purchases permits e-commerce systems to apprehend their alternatives and shopping for conduct.
- Browsing Behavior: Tracking customers’ interactions with product pages, seeking queries, and class navigation affords precious insights into their pastimes and intentions.
- Demographic Information: User demographics along with age, gender, vicinity, and profit level can further refine tips and customize the purchasing experience.
- User Feedback: Incorporating comments from consumer evaluations, scores, and surveys helps e-trade sites first-class-tune their advice algorithms and improve the relevance of guidelines.
The Role of Machine Learning
Machine-gaining knowledge performs a vital function in powering advice algorithms, permitting e-trade structures to continuously optimize and refine their models based on actual-time records. By using strategies including supervised studying, unsupervised getting to know, and reinforcement learning, machine mastering algorithms can examine from beyond interactions and adapt to converting person preferences through the years.
- Supervised Learning: In supervised mastering, algorithms are skilled in classified information, in which every instance is related to a target outcome (e.g., whether or not a person purchased an advocated item). By gaining knowledge from those categorized examples, supervised studying algorithms can expect future results based on new enter statistics.
- Unsupervised Learning: Unsupervised studying strategies, consisting of clustering and dimensionality reduction, are used to perceive hidden patterns and systems in data without express labels. Unsupervised studying algorithms can uncover similarities between products and users, allowing extra accurate hints.
- Reinforcement Learning: Reinforcement gaining knowledge of algorithms learn how to make sequential choices by interacting with an environment and receiving remarks in the form of rewards or consequences. In the context of e-trade advice systems, reinforcement gaining knowledge may be used to optimize long-term consumer engagement and satisfaction.
Conclusion
In the end, advice algorithms lie at the heart of customized purchasing reviews on e-trade websites, leveraging data and device-gaining knowledge to expect customers’ desires and alternatives. By knowledge of the science at the back of these algorithms and their underlying techniques, both consumers and e-trade structures can maximize the cost of personalized pointers in the digital market.
Frequently Asked Questions (FAQ’s)
Ans. E-commerce sites take personal privacy critically and cling to strict information protection rules. Personal information is anonymized and aggregated to make certain individual users can’t be identified.
Ans. Like any machine getting to know the system, recommendation algorithms can exhibit biases if now not well designed and monitored. Biases might also get up from imbalanced facts, algorithmic assumptions, or accidental remark loops.
Ans. Recommendation algorithms are designed to adapt to converting alternatives and developments, inclusive of the introduction of recent or niche merchandise. By continuously gaining knowledge of from personal interactions and remarks, advice structures can discover emerging trends and incorporate them into their tips through the years.
Ans. While advice algorithms can drastically enhance the purchasing revel, they’re no longer without obstacles. Factors including limited person records, sparse interactions, and context-dependent choices can affect the accuracy of recommendations. E-trade systems continually paint to conquer those demanding situations through statistics enrichment, algorithmic enhancements, and consumer remarks mechanisms.
Ans. E-commerce websites use various metrics to assess the performance of advice algorithms, including click-through rate (CTR), conversion rate, average order price (AOV), and client retention. By studying those metrics, e-commerce structures can examine the impact of hints on consumer engagement, satisfaction, and revenue technology.